Fady S. Alnajjar
Research Scientist
List of Research Topics
Topic | Neural synergy: The Future of Motor Function Development and Recovery (2012 ~ ....)
​
Understanding our brain mechanisms, including cognition, emotion, sensation, behavioral adaptation, etc., are essential to gain fundamental knowledge of our neural system. This understanding will not only assist the development and the recovery of our neural system when needed, but also to achieve robust bio-inspired intelligent systems, such as: neuroprosthetics, neurorobotics, etc. In this particular study, we are targeting neuromuscular strategies behind behavioral adaptation and recovery in healthy participants and stroke survivals. Relying on the concept of muscle synergy that defines by low-dimensional signals the synchronization level of several muscles acting for complex motor behavior, we first developed various physiological markers to quantify the motor skill in healthy participants. We then evaluated the markers to classify the motor disabilities of cortical stroke survivors. Three novel markers were yet designed and verified through tasks involving automatic body responses and voluntary actions: 1) the synergy stability index (SSI), which measures the similarities between muscle usage in repeated behaviors and therefore the stability of the neural command. 2) The synergy coordination index (SCI), which measures the overall size of the synergy space required to carry out a movement and therefore the level of coordination between muscles. 3) The synergy dimensionality index (SDI), which measures the number of neural commands sufficient to control the muscles to produce the desired behavior. Currently, we are expanding the project by designing a neuro-feedback model based on sensory and muscle synergies. The model driven by an intelligent robot will aim to assist the development of both skill acquisition and therapeutic approaches, in the hope of making conventional training and rehabilitative systems effortless and more effective. Functional neuroimaging study are planned to be used to track neural level changes along the training period to validate our model.
Topic | Human BodyBalance-like Controller (2011~2012)
​
Neuro modeling is continuously being used to understand the concepts behind various complex biological systems. In this study, a biological inspired computational model that could be used to explore the neuro-sensorimotor basis behind the posture-reflex-response, the essential trigger to support the human balance while standing, is proposed. The model is attempting simulating the rule of the Central Nervous System (CNS) in dealing with the complexity level of the sensorimotor signal flows to perform natural behavior. Our assumption here is that the CNS deals only with a relatively small but valuable amount of data to process useful information. To fulfill this assumption, input\output signals to\from the model is simplified into two hierarchy levels: a low-dimension space (we called synergy weight W) and a high-dimension space (we called neural command C). Thus, leads to a bow-tie-like architecture. The question to be discussed is what type of learning methodology is suitable to fit a model that uses input and output synergies would actually effectively reduce the muscles and sensors redundancies and produces a suitable state of information to construct meaningful coordinated movements. Non-negative matrix factorization (NMF) was used to identify these synergies. Software for interactive musculoskeletal modeling (SIMM) has been used to construct, train and validate the proposed model. The adopted task was the human posture-reflex-response to ground lateral perturbations. Data used in experiment were collected from 12 subjects. Result shows that the proposed model is able to produce meaningful coordinate movements that match experimental data. We believe that our proposed model can offer a scientific approach to the comprehension of the sensorimotor-neural relationship and learning techniques that may suggest various applications for neural rehabilitation.
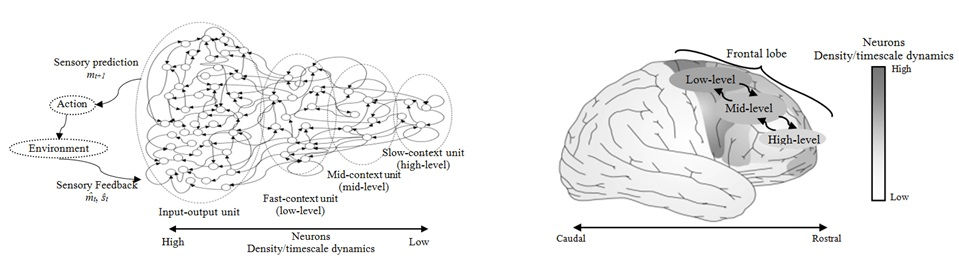
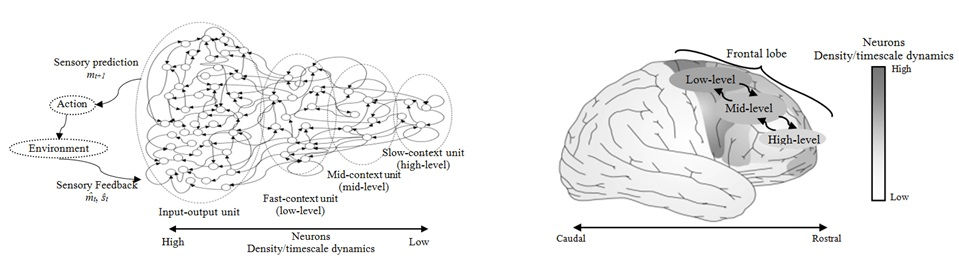
Topic | Static and Dynamic Memory to Simulate Higher-Order Cognitive Tasks (2010~2012)
​
It is widely agreed by the anatomical and neuroimaging studies that the higher order cognitive functions such as cognitive branching, switching, planning, reasoning, and other complex cognitive activities, are the results of the neural dynamics between local regions in the frontal lobe. The underlying mechanisms governing the way of how the macro-level anatomical structures, such as the connectivity between the frontal lobe regions and their local dynamic properties, can be self-organized to obtain such as higher-level tasks, however, not yet been clarified. Although some earlier studies have attempted to localize these tasks with specified areas on the lateral frontal lobe namely the FPC, Mid-PFC, and Pre-PMD, a model is still needed to confirm these finding and to offer a better insight of how the “working memory” is reformed to assist in accomplishing these tasks. To address this issue, we propose a distinct hierarchical Multi-Timescale Recurrent Neural Network model (MTRNN). The utility of this model is that the functional hierarchy is self-organized not only by the force of the layout architecture of the neural connections but also through multi distinct type of neurons, each with different time properties. To verify the validity of the model, a physical experiment was conducted on a humanoid robot in a physical environment. Experimental results suggest that both the neural dynamics and the network layout architecture (i.e., which areas are connected to which) are important factors to reform the working memory that is essential to achieve such as task. This study is a part of study series that intent to construct a neural computational model that in the end aims to attain a Large-Scale Brain Network (LSBN). This proposed study can be considered as a joint research between the synthetic and the empirical studies, which can open a new era for better understanding of brain mechanisms.
The above is just a sample of my research topics. To see more or discuss possible collaborations >>
Topic | BB
Topic | MC

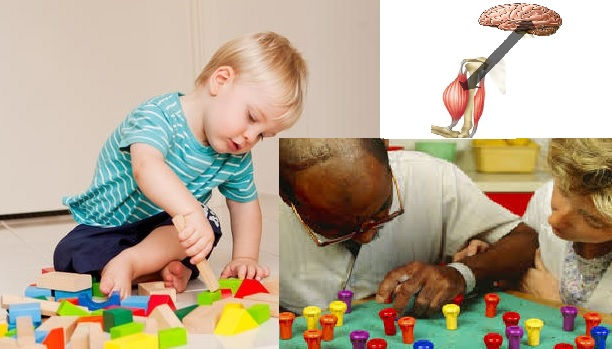

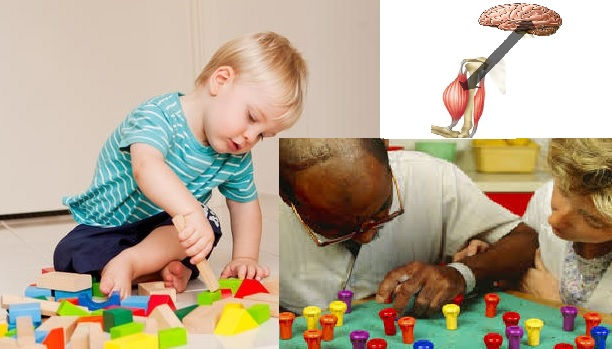
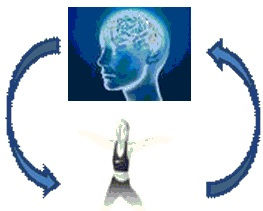

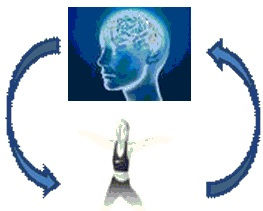

Topic | PhD.
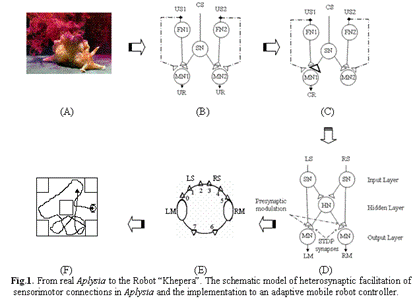

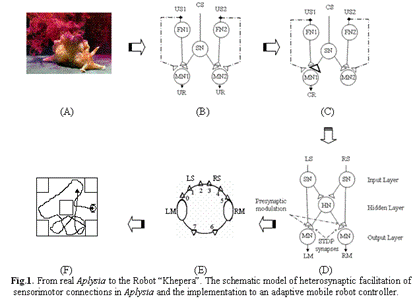

Topic | PhD thesis - Bio-inspired Adaptive Controller for Mobile Robot - abstract (2007~2010)
​
Achieving a bio-inspired adaptive controller for autonomous robot, that resembles living organisms in some specific characteristics, is one of the many challenges that robotics researchers face. Such a controller hopes to come up with robots that have better agility and flexibility, that display a novel functionality, that are more adaptive and intelligent, and that are closer to cope with real world applications. These features are hard or even impossible to be achieved by most of the existing algorithms which mainly designed by the conventional Artificial Neural Network (ANN) (Floreanoand Mattiussi 2008). Such as networks are usually lacked of plasticity: the ability to automatically adapt the agent to unseen and changeable environments, and/or stability: the ability to preserve the agent’s performance in a certain range of adaptation by keeping its past knowledge to enhance its future actions, which are both considered the foundation of any successful autonomous adaptive controller. This thesis, therefore, suggests four different adaptive controllers for physical robot. The proposed controllers hope to overcome the weakness of some of the existing models and attempt to access, to some degree, most of the above listed features.
The first controller, the core of this thesis, is built by the latest generation of ANNs called Spiking NeuralNetwork(SNN). The controller structure and its working mechanism are inspired by the associative facilitation in the withdrawal reflex circuit of the sea slug, “Aplysia”. We called the model, therefore, Aplysia-like Spiking Neural Network (ASNN). The plasticity level of the network is achieved by SpikeTiming-Dependent Plasticity (STDP), which is newly found in natural synapses, and is considered an essential mechanism for learning and memory. The validity of the model to perform navigation and obstacle avoidance tasks on a physical mobile robot in an open environment has been examined by the experiments’ results.
An extension of the controller to cope with advanced tasks of a physical mobile robot is also achieved. A novel controller that binds N number of ASNN, each of which could interact with particular sensory information and produce various motor outputs is proposed at this stage. We called this model MultiAplysia-like Spiking Neural Network (MASNN).
The stability of the controller is also taken into account by proposing an attachable tree-type memory structure. We called it a Dynamic Memory Aplysia-like Spiking Neural Network (DMASNN). Experimental results show that by such a model, the robot can reach to a level of plasticity and stability to survive in highly dynamic environments.
The second controller aims to handle highly complex environments by simplifying them into simple groups of patterns or behaviors, each of which the robot can independently learn and memorize. The controller consists of a Pulse Differentiation Learning Algorithm (PDL) and a Pattern Association NetworkController (PAN-C), where the learning and memory levels take place, respectively.
The third controller intends to achieve a basic level of artificial cognition in a physical mobile robot using a memory-based learning algorithm. In the proposed controller, a novel vision-motor abstraction level that occurred in the robot during its navigation, able to develop a kind of simple anticipation mechanism into its internal world to guide its behavior in the absence of the external inputs.
The fourth controller suggests a new mechanism to develop an incremental learning and memory model; we called a Hierarchical Constructive BackPropagation with Memory (HCBPM). The validity of the model was evaluated in teaching a humanoid robot a group of names (colors). The experimental results indicate the efficiency of the model to build a kind of social learning environment between the user and the robot through real-time interactions. The robot could learn various color names with its different phases, and retrieve these data from its memory to teach another user what it had learned.

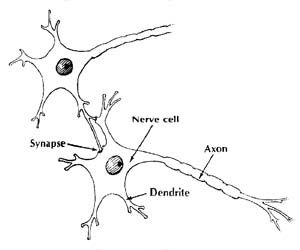
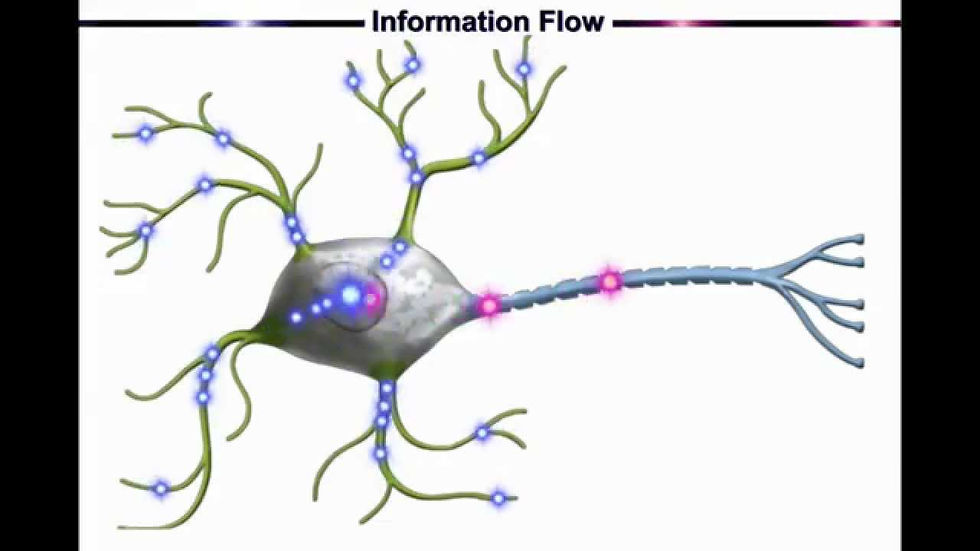


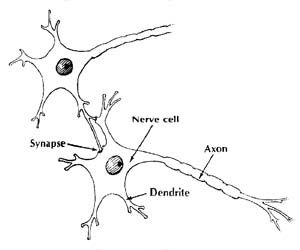
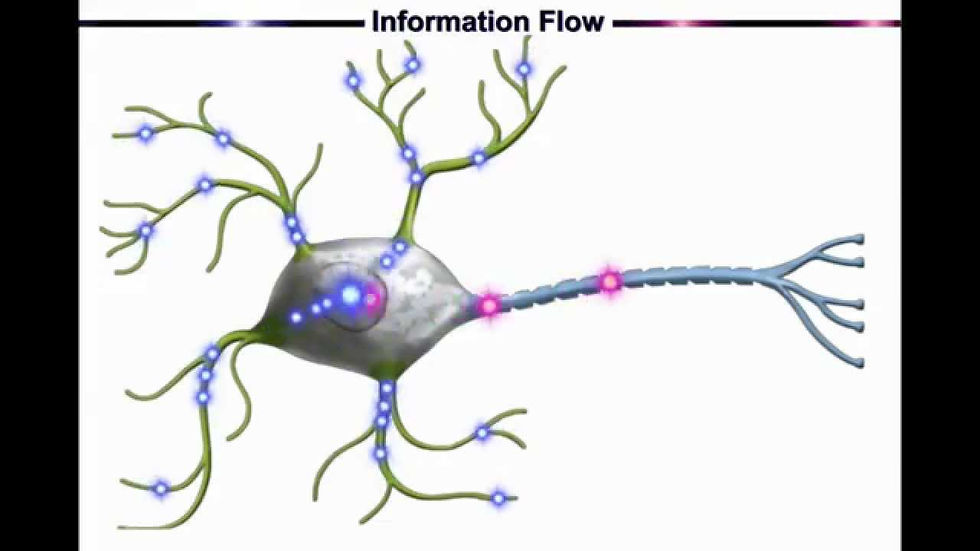

Topic | MS
Topic | Edu
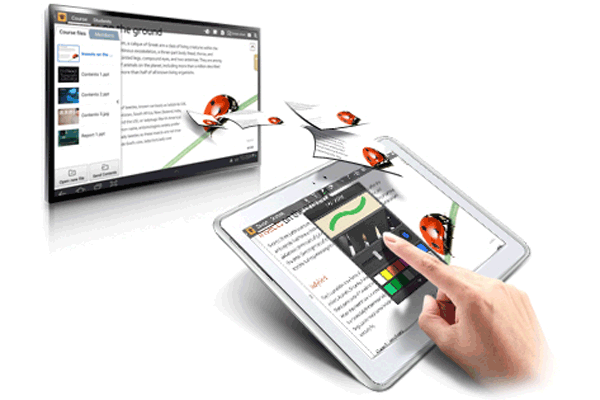
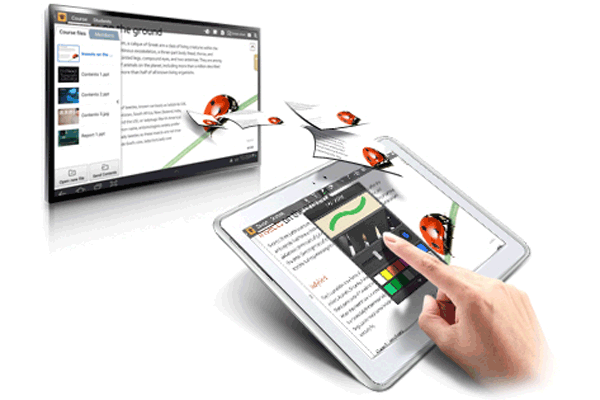
Topic | M.E thesis - Spiking Neural Network (SNN) Model for Robot Navigation system - abstract (2004~2007)
​
This thesis contains two different algorithms that applicable in spiking neural network (SNN) models to develop adaptive controller for autonomous mobile robot.
“Part I” of this thesis introduces the first algorithm (the first project), in which we propose a self-organization algorithm of SNN applicable to autonomous robot for generation of adoptive and goal-directed behavior. In this project, first, we formulated a SNN model whose inputs and outputs were analog and the hidden unites are interconnected each other. Next, we implemented it into a miniature mobile robot Khepera. In order to see whether or not a solution(s) for the given task(s) exists with the SNN, the robot was evolved with the genetic algorithm in the environment. The robot acquired the obstacle avoidance and navigation task successfully, exhibiting the presence of the solution. After that, a self-organization algorithm based on a use-dependent synaptic potentiation and depotentiation at synapses of input layer to hidden layer and of hidden layer to output layer was formulated and implemented into the robot. In the environment, the robot incrementally organized the network and the given tasks were successfully performed. The time needed to acquire the desired adaptive and goal-directed behavior using the proposed self-organization method was much less than that with the genetic evolution, approximately one fifth.
“Part II” includes the abstract and a brief introduction of the main idea of the second project. In which, we capture some of the capabilities of animals’ network structures and functions (e.g. Aplysia) to build a simple SNN structure that can achieve real-work robot tasks. In the experiments with a real robot, such a simple network controller succeeded to adapt the robot in a given environment with a very short time in comparison with that use existing SNN controllers.
From these studies, we exhibited that the SNN is suitable for adaptive controller of autonomous robots. The algorithms developed in this study could be applicable to generate human-like responses in such as humanoid and other man-machine interfaces. In order to expand this work in the future, it is necessary to develop algorithms that can handle a much large number of inputs simultaneously in organized manner.
Topic | Applications for harnessing the current computer technologies towards enhancing the world education (For this work I was invited as a speaker in Education Without Border 2009, March 31~April 1, 2009, Dubai, UAE)
​
Abstract - In recent decades, the computer technology level has been reached to an advanced stage of development. Harnessing such development towards fabricating real applications applicable for enhancing the worldwide education curricula is one of the significant challenges tasks for many groups of researchers. Although there are many works have been done regarding to this issue, with various degree of success, the investigation around such matter is still open. In this short manuscript, we are aiming to highlight four novel ideas that could contribute toward enhancing the common school/university education systems. The ideas were inspired from some recent research theories that can easily applicable and understandable. The first project aims to develop “A ministry/lectures & lecturer/students online interaction systems”, where the lower-layer shows improving in his/her interest when frequent comments are always processed by the higher-layer. The second project presents “A Self-development Planning Advising System (Sd-PAS)”, in which the difficulty of study/vocational decision making for adolescence and fresh graduate students are discussed and positive solution are addressed. The third project illustrates how the self-intelligent and group communication intelligent methodology are different from each other and how these differences can clarify the importance of having frequent diverse student exchange programs. Finally by the fourth project, we introduce the synchronous communication towards a new idea and positive conclusion from organized group meeting.
We believe that the proposed ideas in this study can be successfully applied to a broad range of applications and it could address new methodologies of future technologies that can be applied into our education system.
Topic | NS




Topic | NS
Topic | Prosthetic arm: A Tool to Learn, or "a Body-Part" to Adapt
​
We can learn how to drive a car or ride a bike; we can also learn how to use a scissor or screwdriver. Nevertheless, we don't need to learn how much we need to rotate our wrist to gently grip a cup or a pencil from a front desk, nor how much you have to move the muscles in your arm to scratch your back. Our brain can carefully distinguish between the tools that it should learn how to use, and the body parts that it has to adapt to function it. Most of the commercial prosthetic devices are designed to be a tool, thus the users need to learn how to control. We believe that building a body-part-like prosthetic arm will force the brain to adapt to it, not to learn how to use it as a tool (See Figure 1 for the difference between the two principles). Our research direction can guarantee more sophisticated and complex, yet, easy to control prosthetic devices.
From our previous publication (1), figure 1 shows A conseptual design of neural sensorimotor synergy system. (A) The acquired sensory signals and inferred artificial sensory synergies contribute to the sensory synergy. (B) The prosthesis controller computes motor commands based on the artificial sensory synergies. (C) The motor commands are recruited at the activators of the prosthesis in order to enable the wearer to control the motors. The motion of an artificial wrist joint adapts to the motion of the disarticulated arm in order to facilitate grasping and manipulation tasks.
​
References:
(1) Alnajjar, Fady, Matti Itkonen, Vincent Berenz, Maxime Tournier, Chikara Nagai, and Shingo Shimoda. “Sensory Synergy as Environmental Input
Integration.” Frontiers in Neuroscience 8 (January 13, 2015). doi:10.3389/fnins.2014.00436
​
​